In this Audio Overview, two AI hosts dive into the world of NotebookLM updates.
2024: A year of extraordinary progress and advancement in AI
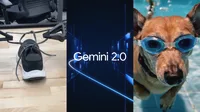
As we move into 2025, we wanted to take a moment to recognize the astonishing progress of the last year. From new Gemini models built for the agentic era and empowering creativity, to an AI system that designs novel, high-strength protein binders, AI–enabled neuroscience and even landmark advances in quantum computing, we’ve been boldly and responsibly advancing the frontiers of artificial intelligence and all the ways it can benefit humanity.
As we and our colleagues wrote two years ago in an essay titled Why we focus on AI:
“Our approach to developing and harnessing the potential of AI is grounded in our founding mission — to organize the world’s information and make it universally accessible and useful — and it is shaped by our commitment to improve the lives of as many people as possible.”
This remains as true today as it was when we first wrote it.
In this 2024 Year-in-Review post, we look back on a year's worth of extraordinary progress in AI, made possible by the many incredible teams across Google, that helped deliver on that mission and commitment — progress that sets the stage for more to come this year.
Relentless innovation in models, products and technologies
2024 was a year of experimenting, fast shipping, and putting our latest technologies in the hands of developers.
In December 2024, we released the first models in our Gemini 2.0 experimental series — AI models designed for the agentic era. First out of the gate was Gemini 2.0 Flash, our workhorse model, followed by prototypes from the frontiers of our agentic research including: an updated Project Astra, which explores the capabilities of a universal AI assistant; Project Mariner, an early prototype capable of taking actions in Chrome as an experimental extension; and Jules, an AI-powered code agent. We're looking forward to bringing Gemini 2.0’s powerful capabilities to our flagship products — in Search, we’ve already started testing in AI Overviews, which are now used by over a billion people to ask new types of questions.
We also released Deep Research, a new agentic feature in Gemini Advanced that saves people hours of research work by creating and executing multi-step plans for finding answers to complicated questions; and introduced Gemini 2.0 Flash Thinking Experimental, an experimental model that explicitly shows its thoughts.
These advances followed swift progress earlier in the year, from incorporating Gemini’s capabilities into more Google products to the release of Gemini 1.5 Pro and Gemini 1.5 Flash — a model optimized for speed and efficiency. 1.5 Flash’s compact size made it more cost-efficient to serve, and in 2024 it became our most popular model for developers.
And we improved and updated AI Studio, which provides a host of resources for developers. It is now available as a progressive web app (PWA) that can be installed on desktop, iOS and Android.
Notably, it’s been exciting to see the public reception to several new features for NotebookLM, such as Audio Overviews, which can take uploaded source material and produce a “deep dive” discussion between two AI hosts.
More natural and intuitive handling of speech input and output remains at the core of several of our products: Gemini Live, Project Astra, Journey Voices and YouTube’s auto dubbing.
Continuing our long history of contributing innovations to the open community — such as with Transformers, TensorFlow, BERT, T5, JAX, AlphaFold and AlphaCode — we released two new models from Gemma, our state-of-the-art open model built from the same research and technology used to create the Gemini models. Gemma outperformed similarly sized open models on capabilities like question answering, reasoning, math / science and coding. And we released Gemma Scope, which provides tools that help researchers understand the inner workings of Gemma 2.
We also continued to improve the factuality of our models and minimize hallucinations. In December, for example, we published FACTS Grounding, a new benchmark — based on collaboration between Google DeepMind, Google Research and Kaggle — for evaluating how accurately large language models ground their responses in provided source material and avoid hallucinations.
The FACTS Grounding dataset comprises 1,719 examples, each carefully crafted to require long-form responses grounded in the context document provided.
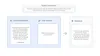
We tested leading LLMs using FACTS Grounding, launched the FACTS leaderboard on Kaggle and are proud that Gemini 2.0 Flash Experimental, Gemini 1.5 Flash and Gemini 1.5 Pro currently have the three highest factuality scores, with gemini-2.0-flash-exp at 83.6%.
Moreover, we improved underlying ML efficiency through pioneering techniques like blockwise parallel decoding, improved confidence-based deferral and speculative decoding that reduce the inference times of LLMs, allowing them to generate responses more quickly. These improvements are used across Google products and set a standard throughout the industry.
Combining AI with sport, in March we released TacticAI, an AI system for football tactics that can provide experts with tactical insights, particularly on corner kicks.
Underlying all of our models and products is our ongoing commitment to research leadership. Indeed, in a 2010-2023 WIPO survey of citations for papers on Generative AI, Google including Google Research and Google DeepMind’s citations were more than double the second-most cited institution.
This WIPO graph, based on January 2024 data from The Lens, illustrates more than a decade’s worth of Alphabet’s generative AI scientific publication efforts.
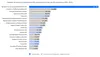
Finally, progress was made with Project Starline, our “magic window” technology project that enables friends, families and coworkers to feel like they’re together from any distance. We partnered with HP to start commercialization, with the goal of enabling it directly from video conferencing services like Google Meet and Zoom.
Empowering creative vision with generative AI
We believe AI holds great potential to enable new forms of creativity, democratize creative output and help people express their artistic visions. This is why last year we introduced a series of updates across our generative media tools, covering images, music and video.
At the start of 2024, we introduced ImageFX, a new generative AI tool that creates images from text prompts, and MusicFX, a tool for creating up-to-70-second audio clips also based on text prompts. At I/O, we shared an early preview of MusicFX DJ, a tool that helps bring the joy of live music creation to more people. In October, we collaborated with Jacob Collier on making MusicFX DJ simpler to use, especially for new or aspiring musicians. And we updated our music AI toolkit Music AI Sandbox, and evolved our Dream Track experiment which allowed U.S. creators to explore a range of genres and prompts that generate instrumental soundtracks with powerful text-to-music models.
Later in 2024, we released state-of-the-art updates to our image and video models: Veo 2 and Imagen 3. As our highest quality text-to-image model, Imagen 3 is capable of generating images with even better detail, richer lighting and fewer distracting artifacts than our previous models; while Veo demonstrated an improved understanding of real-world physics and the nuances of human movement and expression alongside its overall attention-to-detail and realism.
Veo represents a significant step forward in high-quality video generation.
Research in this field continued apace. We explored ways to use AI to improve editing, for example by using it to control of attributes like transparency, roughness or other physical properties of objects:
In these examples of AI editing with synthetic data generation, Input shows a novel, held-out image the model has never seen before. Output shows the model output, which successfully edits material properties.
In the field of audio generation, we announced improvements to video-to-audio (V2A) technology, which can generate dynamic soundscapes through natural language text prompts based on on-screen action. This technology is pairable with AI-created video through Veo.
Games are an ideal environment for creative exploration of new worlds, as well as training and evaluating embodied agents. In 2024, we introduced Genie 2, a foundation world model capable of generating an endless variety of action-controllable, playable 3D environments for training and evaluating embodied agents. This followed the introduction of SIMA, a Scalable Instructable Multiworld Agent that can follow natural-language instructions to carry out tasks in a variety of video game settings.
The architecture of intelligence: advances in robotics, hardware and computing
As our multimodal models become more capable and gain a better understanding of the world and its physics, they are making possible incredible new advances in robotics and bringing us closer to our goal of ever-more capable and helpful robots.
With ALOHA Unleashed, our robot learned to tie a shoelace, hang a shirt, repair another robot, insert a gear and even clean a kitchen.
At the beginning of the year, we introduced AutoRT, SARA-RT and RT-Trajectory, extensions of our Robotics Transformers work intended to help robots better understand and navigate their environments, and make decisions faster. We also published ALOHA Unleashed, a breakthrough in teaching robots on how to use two robotic arms in coordination, and DemoStart, which uses a reinforcement learning algorithm to improve real-world performance on a multi-fingered robotic hand by using simulations.
Robotic Transformer 2 (RT-2) is a novel vision-language-action model that learns from both web and robotics data.
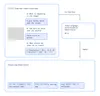
Beyond robotics, our AlphaChip reinforcement learning method for accelerating and improving chip floorplanning is transforming the design process for chips found in data centers, smartphones and more. To accelerate adoption of these techniques, we released a pre-trained checkpoint to enable external parties to more easily make use of the AlphaChip open source release for their own chip designs. And we made Trillium, our sixth-generation and most performant TPU to date, generally available to Google Cloud customers. Advances in computer chips have accelerated AI. And now, AI can return the favor.
AlphaChip can learn the relationships between interconnected chip components and generalize across chips, letting AlphaChip improve with each layout it designs.
Our research also focused on correcting the errors in the physical hardware of today's quantum computers. In November, we launched AlphaQubit, an AI-based decoder that identifies quantum computing errors with state-of-the-art accuracy. This collaborative work brought together Google DeepMind’s ML knowledge and Google Research’s error correction expertise to accelerate progress on building a reliable quantum computer. In tests, it made 6% fewer errors than tensor network methods and 30% fewer errors than correlated matching.
Then in December, the Google Quantum AI team, part of Google Research, announced Willow, our latest quantum chip which can perform in under five minutes a benchmark computation that would take one of today’s fastest supercomputers 10 septillion years. Willow can reduce errors exponentially as it scales up using more qubits. In fact, it used our quantum error correction to cut the error rate in half, solving a 30+ year challenge known in the field as “below threshold.” This leap forward won the Physics Breakthrough of the Year award.
Willow has state-of-the-art performance across a number of metrics.
Uncovering new solutions: progress in science, biology and mathematics
We continued to push the envelope on accelerating scientific progress with AI-based approaches, releasing a series of tools and papers this year that showed just how useful and powerful a tool AI is for advancing science and mathematics. We're sharing a few highlights.
In January, we introduced AlphaGeometry, an AI system engineered to solve complex geometry problems. Our updated version, AlphaGeometry 2, and AlphaProof, a reinforcement-learning-based system for formal math reasoning, achieved the same level as a silver medalist in July 2024’s International Mathematical Olympiad.
AlphaGeometry 2 solved Problem 4 in July 2024’s International Mathematical Olympiad within 19 seconds after receiving its formalization. Problem 4 asked to prove the sum of ∠KIL and ∠XPY equals 180°.
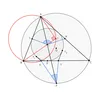
In collaboration with Isomorphic Labs, we introduced AlphaFold 3, our latest model which predicts the structure and interactions of all of life’s molecules. By accurately predicting the structure of proteins, DNA, RNA, ligands and more, and how they interact, we hope it will transform our understanding of the biological world and drug discovery.
AlphaFold 3’s capabilities come from its next-generation architecture and training that now covers all of life’s molecules.
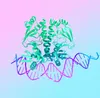
We made several key developments in protein-shaping. We announced AlphaProteo, an AI system for designing novel, high-strength protein binders. AlphaProteo can lead to the discovery of new drugs, the development of biosensors and improve our understanding of biological processes.
AlphaProteo can generate new protein binders for diverse target proteins.
In collaboration with Harvard’s Lichtman Lab and others, we produced a nano-scale mapping of a piece of the human brain at a level of detail never previously achieved, and made it publicly available for researchers to build on. This follows a decade of working to advance our understanding of connectomics, with earlier work on fly brain and mouse brain connectomics now giving way to the larger scale and more complex human brain connectomics.
In the deepest layer of the cortex, clusters of cells tend to occur in mirror-image orientation to one another, as shown in this brain mapping project.
Then in late November, as part of a broader effort to expand and deepen public dialogue around science and AI, we co-hosted the AI for Science Forum with the Royal Society, which convened scientists, researchers, governmental leaders and executives to discuss key topics like cracking the protein structure prediction challenge, mapping the human brain and saving lives through accurate forecasting and spotting wildfires. We hosted a Q&A with the four Nobel Laureates in attendance at the forum, Sir Paul Nurse, Jennifer Doudna, Demis Hassabis and John Jumper, which is available to listen to via the Google DeepMind podcast.
This was also a landmark year for another reason: Demis Hassabis and John Jumper, along with David Baker, were awarded the 2024 Nobel Prize® in Chemistry for their work on AlphaFold 2. As the Nobel committee recognized, their work:
"[H]as opened up completely new possibilities to design proteins that have never been seen before, and we now have access to predicted structures of all 200 million known proteins. These are truly great achievements."
It was also exciting to see the 2024 Nobel Prize® in Physics awarded to recently retired long-time Googler Geoffrey Hinton (along with John Hopfield), "for foundational discoveries and inventions that enable machine learning with artificial neural networks.”
The Nobels followed additional recognitions for Google including the NeurIPS 2024 Test of Time Paper Awards for Sequence to Sequence Learning with Neural Networks and Generative Adversarial Nets, and the Beale—Orchard-Hays Prize, which was awarded to a collaborative team of educators and Google professionals for groundbreaking work on Primal-Dual Linear Programming (PDLP). (PDLP, now part of Google OR Tools, helps solve large-scale linear programming problems with real-world applications from data center network traffic engineering to container shipping optimization.)
AI for the benefit of humanity
This year, we made a number of product advances and published research that showed how AI can benefit people directly and immediately, ranging from preventative and diagnostic medicine to disaster readiness and recovery to learning.
In healthcare, AI holds the promise of democratizing quality of care in key areas, such as early detection of cardiovascular disease. Our research demonstrated how using a simple fingertip device that measures variations in blood flow, combined with basic metadata, can predict heart health risks. We built on previous AI-enabled diagnostic research for tuberculosis, demonstrating how AI models can be used for accurate TB screenings in populations with high rates of TB and HIV. This is important to reducing the prevalence of TB (more than 10 million people fall ill with it each year), as roughly 40% of people with TB go undiagnosed.
On the MedQA (USMLE-style) benchmark, Med-Gemini attains a new state-of-the-art score, surpassing our prior best (Med-PaLM 2) by a significant margin of 4.6%.
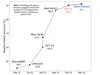
Our Gemini model is a powerful tool for professionals generally, but our teams are also working to create fine-tuned models for other domains. For example, we introduced Med-Gemini, a new family of next-generation models that combine training on de-identified medical data with Gemini’s reasoning, multimodal and long-context abilities. On the MedQA US Medical Licensing Exam (USMLE)-style question benchmark, Med-Gemini achieves a state-of-the-art performance of 91.1% accuracy, surpassing our prior best of Med-PaLM 2 by 4.6% (shown above).
We are exploring how machine learning can help medical fields struggling with access to imaging expertise, such as radiology, dermatology and pathology. In the past year, we released two research tools, Derm Foundation and Path Foundation, that can help develop models for diagnostic tasks, image indexing and curation and biomarker discovery and validation. We collaborated with physicians at Stanford Medicine on an open-access, inclusive Skin Condition Image Network (SCIN) dataset. And we unveiled CT Foundation, a medical imaging embedding tool used for rapidly training models for research.
With regard to learning, we explored new generative AI tools to support educators and learners. We introduced LearnLM, our new family of models fine-tuned for learning and used it to enhance learning experiences in products like Search, YouTube and Gemini; a recent report showed LearnLM outperformed other leading AI models. We also made it available to developers as an experimental model in AI Studio. Our new conversational learning companion, LearnAbout, uses AI to help you dive deeper into any topic you’re curious about, while Illuminate lets you turn content into engaging AI-generated audio discussions.
In the fields of disaster forecasting and preparedness, we announced several breakthroughs. We introduced GenCast, our new high-resolution AI ensemble model, which improves day-to-day weather and extreme events forecasting across all possible weather trajectories. We also introduced our NeuralGCM model, able to simulate over 70,000 days of the atmosphere in the time it would take a physics-based model to simulate only 19 days. And GraphCast won the 2024 MacRobert Award for engineering innovation.
This selection of GraphCast’s predictions rolling across 10 days shows specific humidity at 700 hectopascals (about 3 kilometers above surface), surface temperature and surface wind speed.
We also improved our flood forecasting model to predict flooding seven days in advance (up from five) and expanded our riverine flood forecasting coverage to 100 countries and 700 million people. This marks a significant milestone in a multi-year initiative that Google Research embarked on in 2018.
Our flood forecasting model is now available in over 100 countries (left), and we now have “virtual gauges” for experts and researchers in more than 150 countries, including countries where physical gauges are not available.
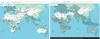
AI can also help with wildfire detection and mitigation, which is especially top of mind given the devastation in California. Our Wildfire Boundary Maps capabilities are now available in 22 countries. Alongside leading wildfire authorities, Google Research also created FireSat, a constellation of satellites that can detect and track wildfires as small as a classroom (roughly 5x5 meters) within 20 minutes.
And we continued building on our commitment to making more information more accessible to more people, expanding Google Translate with 110 new languages, including Cantonese, Papua New Guinea’s Tok Pisin, N’Ko from West Africa and Manx from the Isle of Man. Google Translate — which now supports over 240 languages — can help people overcome barriers to information, knowledge and opportunity.
These new languages in Google Translate represent more than 614 million speakers, opening up translations for around 8% of the world’s population.
Helping set the standard in responsible AI
We furthered our industry-leading research in AI safety, developing new tools and techniques and integrating these advances into our latest models. We’re committed to working with others to address risks.
We continued researching misuse, conducting a study that found the two most common types of misuse were deep fakes and jailbreaks. In May, we introduced The Frontier Safety Framework, which established protocols for identifying the emerging capabilities of our most advanced AI models, and launched our AI Responsibility Lifecycle framework to the public. In October, we expanded our Responsible GenAI Toolkit to work with any LLM, giving developers more tools to build AI responsibly.
And, among our other efforts, we released a paper this year on The Ethics of Advanced AI Assistants that examined and mapped the new technical and moral landscape of a future populated by AI assistants, and characterized the opportunities and risks society might face.
We expanded SynthID’s capabilities to watermarking AI-generated text in the Gemini app and web experience, and video in Veo. To help increase overall transparency online, not just with content created by Google gen AI tools, we also joined the Coalition for Content Provenance and Authenticity (C2PA) as a steering committee member and collaborated on a new, more secure version of the technical standard, Content Credentials.
When there’s a range of different tokens to choose from, SynthID can adjust the probability score of each predicted token, in cases where it won’t compromise the quality, accuracy and creativity of the output.
Beyond LLMs, we shared our approach to biosecurity for AlphaFold 3. We also worked with industry partners to launch the Coalition for Secure AI (CoSAI), and we participated in the AI Seoul Summit, as a way of building and contributing to an international consensus and a common, coordinated approach to governance.
As we develop new technologies like AI agents, we’ll continue to encounter new questions around safety, security and privacy. Guided by our AI Principles, we are deliberately taking an exploratory and gradual approach to development, conducting research on multiple prototypes, iteratively implementing safety training, working with trusted testers and external experts and performing extensive risk assessments and safety and assurance evaluations.
Looking ahead to 2025
2024 was a productive year, and a very exciting time for groundbreaking new products and research in AI. We made a great deal of progress and we’re even more excited about the year ahead.
As we continue to produce groundbreaking AI research in the fields of products, science, health, creativity and more, it becomes increasingly important to think deeply about how and when it should be deployed. By continuing to prioritize responsible AI practices and fostering collaboration, we’ll play an important role in building a future where AI benefits humanity.